What We Do
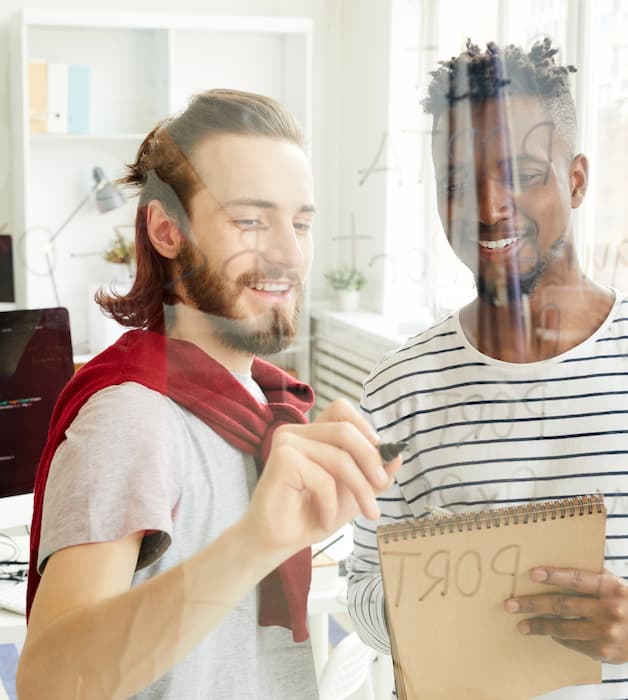
Who We Are
NobleProg is an international training and consultancy group, specializing in custom, live instructor-led training on the newest technologies.
About UsPopular Courses
Course Catalogue
- 3D Modeling
- Artificial Intelligence (AI)
- Augmented Reality (AR)
- Application Performance Management (APM)
- Automotive
- Application Server
- Big Data
- Blockchain
- BPM
- Containers and Virtual Machines (VMs)
- Cloud Computing
- Content Management System (CMS)
- Customer Relationship Management (CRM)
- Cyber Security
- Computer Graphics
- Configuration Management
- Collaboration
- Database
- Data Analysis
- DevOps
- Distributed Systems
- Desktop Publishing (DTP)
- Data Science
- Data Management
- DaDesktop
- Enterprise Resource Planning (ERP)
- Enterprise Architecture
- Embedded Systems
- Enterprise Asset Management (EAM)
- Finance
- Game Development
- Graph Computing
- Graphic Design
- Internet of Things (IoT)
- Industrial Automation
- ISO Standards
- Linux
- Machine Learning
- MLOps
- Mobile Development
- Microservices
- Mobile Networks
- Marketing
- Management
- Microsoft
- Networking
- Operating Systems (OS)
- OMG
- Programming
- Project Management
- Robotic Process Automation (RPA)
- Robotics
- Statistics
- Software Engineering
- Search Engines
- Service-Oriented Architecture (SOA)
- Soft Skills
- User Experience (UX) Design
- Virtual Reality (VR)
- Version Control Systems
- Video Streaming
- Web Development
- Web Services
- Web Server
- Windows OS